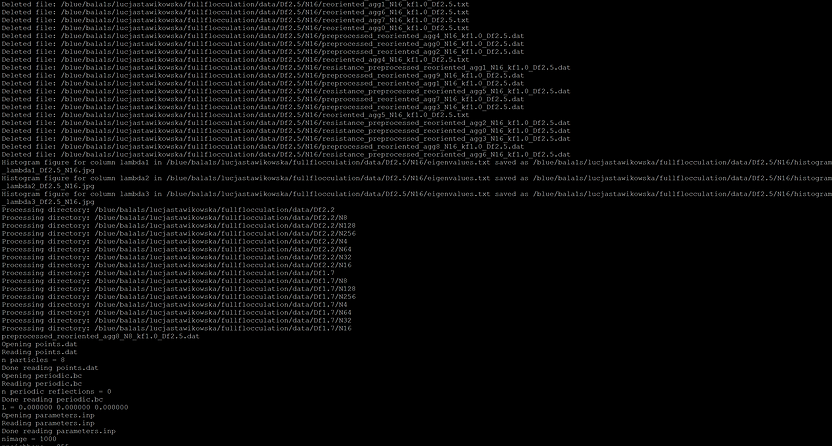

Towards A Digital Twin of the Flocculation Process
University of Florida
Principal Investigator: Dr. Balachandar
UF Department of Mechanical and Aerospace Engineering
Project Start Date: November 2023
Research Focus
In various environmental and engineering applications, small particles suspended in fluid aggregate to form flocculate particle clusters. These particle aggregates can be observed in the smoke from a fire, or in the sediment deposits of a river delta. The shape and size of these particles directly influence their behavior. For example, the shape and size of soot particles determines how quickly they settle after an explosion.
The formation of the aggregate particles begins with smaller sub-particles of roughly the same size, called monomers. The monomers join with each other to form dimers which then join, and so on until the aggregate particle cluster is formed. The monomers and flocs come together by Brownian motion and turbulent shear. To classify the aggregate particles, they will be organized by their number of monomers and their fractal dimension. The number of monomers determines the size, and the fractal dimension describes the shape of the particle, with values of 1.0, 2.0, and 3.0 corresponding to a linear chain, a plane, and a sphere of monomers, respectively.
The current issue with understanding these flocculate particles comes from the oversimplification of their shape. Presently, the general approach is to treat the aggregates as spheres, regardless of their actual shape. This is an issue for several reasons. Considering the shape of the floc is essential to understanding the hydrodynamic force and torque exerted on it by surrounding flow, such an oversimplification will lead to inaccuracies. For example, the simplification method cannot distinguish between the force on a linear chain of flocs aligned with the flow and one that is perpendicular to it. Additionally, it cannot predict the torque on the floc and the resulting motion. Lastly, it does not account for the fact that certain hydrodynamic forces experienced by the flocs can be strong enough to break them apart.
Project Responsibilities
Due to their small size, the interaction between the flocs and the surrounding fluid can be accurately calculated. The force on each monomer particle when the floc is in steady motion can be calculated using Stokes flow theory. Potential flow theory can be used to calculate the force on each monomer when the floc is subject to acceleration or rotational motion. While it is possible to write a code which accurately calculates the forces on each monomer given the shape, size, and orientation of the floc, such a calculation is computationally expensive and impractical when applied to practical flows and large numbers of flocs.
This project aims to use emergent artificial intelligence techniques to calculate the force and torque on particle aggregates with a specified shape and size. Given the wide variety of input parameters, the use of AI will increase the efficiency of the process. We plan to make a machine learning (ML) model which will predict the force and torque on a floc more efficiently and accurately than presently used techniques. The ML model will then be used in a digital twin of the flocculation process and will enable the implementation of virtual experiments concerning how monomer-laden flow becomes a suspension of flocculate particles. The benefit of artificial intelligence is that it will allow us to study the interactions of billions of aggregate particles in a cost-effective and accurate way without the need for inexact approximations. This digital twin will allow us to understand many important physical processes which have yet to be fully understood.
Research History
University of Florida
Center for Compressible Multiphase Turbulence
November 2023 - Present
Florida Atlantic University
BioRobotics Lab
May 2021 - May 2023
Working under Dr. Balachandar to calculate the added mass of nanoparticles with varying number of nodules and fractal dimensions, for various applications in the fluid and particle dynamics fields.
Developed a flexible magnetic sensor array combined with an artificial neural network to classify torsion in prosthetic device applications with 98% accuracy. Presented research findings at four national and state conferences to a total of 350 people over the course of two years.
L. Stawikowska and E. Engeberg. (2023, May 19). Flexible Magnetic Skin Sensor Array for Torsion Perception [Paper presentation]. FCRAR 2023, Tampa, FL, United States. https://doi.org/10.5038/SWID5066